New blog posts
Warum GoodWe in Leipzig die Vorreiterrolle für erneuerbare Energien einnimmt
14 September, 2024 by Evionyx Solar
Die Zukunft der Energiegewinnung ist grün,...

Find Old Tractors at Affordable Rates to Enhance Your Farm’s Productivity
13 September, 2024 by tractor factory
Are you a farmer looking to boost your...

Find Old Tractors at Affordable Rates to Enhance Your Farm’s Productivity
13 September, 2024 by tractor factory
Are you a farmer looking to boost your...
Web Directory
Machine Learning Operations (MLO): Optimizing AI Deployment and Management
Posted on 27 February, 2024 by RUCKUS Networks
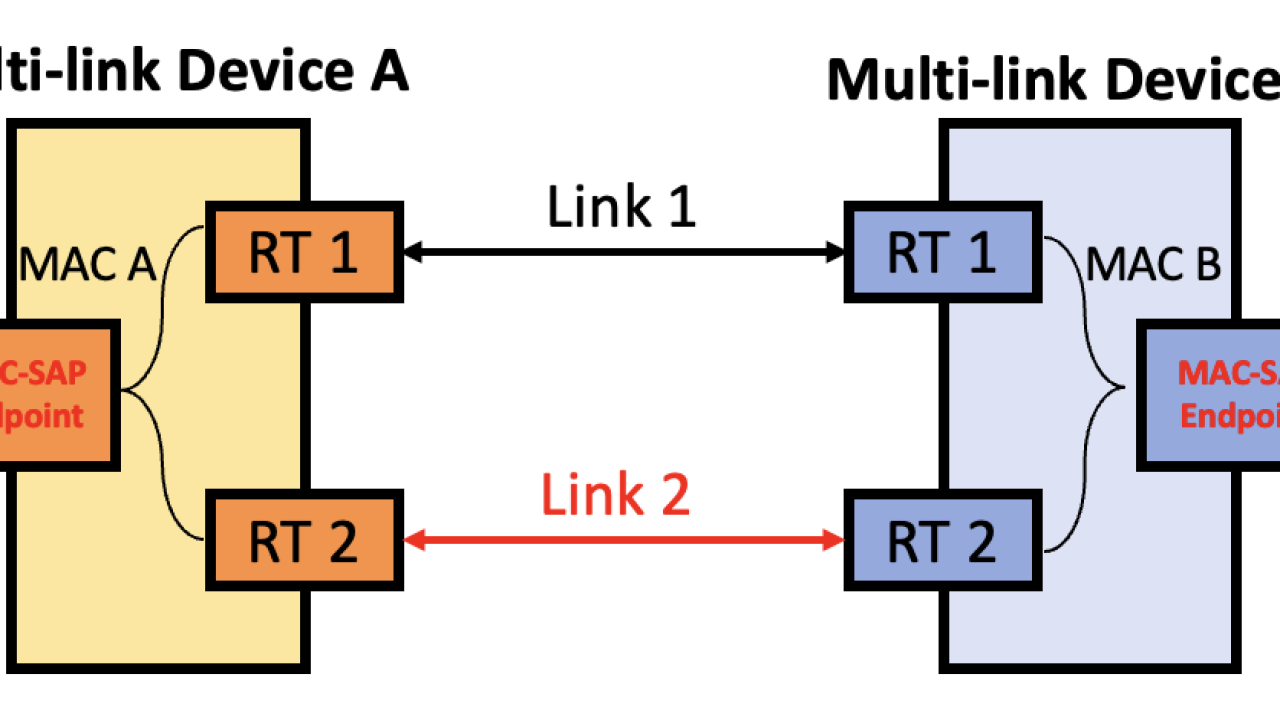
Machine Learning Operations (MLO) refers to the practices and tools used to streamline the deployment, management, and monitoring of machine learning models in production environments. As organizations increasingly adopt artificial intelligence (AI) and machine learning (ML) for various applications, MLO becomes crucial for ensuring efficient, scalable, and reliable AI operations. Let's delve into the significance of MLO, its key components, benefits, and how it empowers organizations to harness the full potential of AI technologies.
The Significance of Machine Learning Operations (MLO):
Scalable Model Deployment:
MLO enables organizations to deploy machine learning models at scale, whether for customer-facing applications, internal processes, or IoT devices.
It ensures that models are integrated seamlessly into existing systems, allowing for rapid deployment and updates.
Model Monitoring and Management:
MLO provides tools for monitoring model performance, detecting drift, and managing model versions.
Organizations can track model accuracy, identify anomalies, and retrain models as needed to maintain optimal performance.
Collaboration and Efficiency:
MLO platforms facilitate collaboration between data scientists, engineers, and operations teams.
It streamlines workflows, from model development to deployment, reducing friction and accelerating the time to market for AI initiatives.
Cost Optimization:
By optimizing resource allocation and automating repetitive tasks, MLO helps organizations reduce the costs associated with managing machine learning models.
It ensures efficient use of computing resources and minimizes downtime due to model errors or failures.
Compliance and Governance:
MLO platforms often include features for ensuring regulatory compliance and maintaining governance standards.
Organizations can track model performance against established benchmarks and ensure adherence to data privacy regulations.
Key Components of Machine Learning Operations (MLO):
Model Training and Development:
MLO encompasses the entire lifecycle of machine learning models, starting from data preparation and feature engineering to model training and validation.
Data scientists use MLO platforms to experiment with different algorithms, hyperparameters, and datasets to develop accurate models.
Model Deployment and Serving:
MLO platforms provide tools for deploying trained models into production environments.
These platforms ensure that models are served efficiently, handling prediction requests from applications or APIs.
Monitoring and Logging:
MLO platforms include monitoring capabilities to track model performance metrics, such as accuracy, latency, and throughput.
Logs and metrics generated by the deployed models help teams identify issues, drift, or anomalies in real-time.
Model Versioning and Management:
Version control is crucial in MLO to track changes to models, datasets, and configurations.
MLO platforms allow for easy model versioning, rollback to previous versions, and comparison of model performance over time.
Automated Pipelines:
MLO platforms often include automated pipelines for data preprocessing, model training, evaluation, and deployment.
These pipelines streamline the workflow, ensuring consistency and reproducibility in model development and deployment.
Benefits of Machine Learning Operations (MLO):
Improved Model Performance:
MLO enables continuous monitoring and retraining of models, leading to improved accuracy and relevance over time.
Models can adapt to changing data patterns and maintain peak performance in dynamic environments.
Faster Time to Market:
With streamlined workflows and automated processes, MLO accelerates the deployment of machine learning models.
Organizations can quickly iterate on models, validate hypotheses, and launch new AI-powered applications.
Reduced Downtime and Costs:
Proactive monitoring and automated alerts in MLO platforms help detect issues early, reducing downtime and preventing costly disruptions.
Efficient resource utilization and optimization lead to cost savings in computing resources and operational expenses.
Enhanced Collaboration:
MLO fosters collaboration between data science, engineering, and operations teams, breaking down silos and improving communication.
Cross-functional teams can work together seamlessly to deliver successful AI initiatives.
Scalability and Flexibility:
MLO platforms are designed to scale with the growing demands of AI applications.
Organizations can easily scale their model deployments, handle increasing data volumes, and adapt to evolving business needs.
Applications of Machine Learning Operations (MLO):
Recommendation Systems:
MLO is used to deploy and manage recommendation algorithms in e-commerce platforms, streaming services, and content platforms.
These systems continuously learn from user interactions to provide personalized recommendations.
Predictive Maintenance:
In industries such as manufacturing and IoT, MLO is used for predictive maintenance of equipment.
Models predict when machines are likely to fail, allowing for proactive maintenance to prevent costly downtime.
Fraud Detection:
MLO platforms are employed in financial services for real-time fraud detection.
Models analyze transaction data to identify suspicious activities and trigger alerts for further investigation.
Healthcare Diagnostics:
In healthcare, MLO supports diagnostic imaging, such as MRI or X-ray analysis.
Models assist radiologists in detecting anomalies and providing more accurate diagnoses.
Natural Language Processing (NLP):
MLO is integral to NLP applications, including chatbots, sentiment analysis, and language translation.
Models process and analyze text data to extract insights and enable automated interactions.
Case Study: Retail Recommendation System
Company ABC, a retail giant, implemented MLO for its recommendation system:
Model Development: Data scientists used MLO platforms to develop and train recommendation algorithms based on customer behavior and preferences.
Model Deployment: The trained models were deployed using MLO tools, integrated into the company's e-commerce platform.
Continuous Monitoring: MLO provided real-time monitoring of model performance, tracking user engagement and conversion rates.
For more info. visit us:
https://latestsms.in/funny-good-morning-sms.htm
24 July, 2024
https://www.cargoes.com/rostering-system
23 November, 2022
https://www.cargoes.com/rostering-system
92474 Views
http://aptrondelhi.in/
26513 Views