New blog posts
Warum GoodWe in Leipzig die Vorreiterrolle für erneuerbare Energien einnimmt
14 September, 2024 by Evionyx Solar
Die Zukunft der Energiegewinnung ist grün,...

Find Old Tractors at Affordable Rates to Enhance Your Farm’s Productivity
13 September, 2024 by tractor factory
Are you a farmer looking to boost your...

Find Old Tractors at Affordable Rates to Enhance Your Farm’s Productivity
13 September, 2024 by tractor factory
Are you a farmer looking to boost your...
Web Directory
Benefits of ML in Network Monitoring
Posted on 30 April, 2024 by RUCKUS Networks
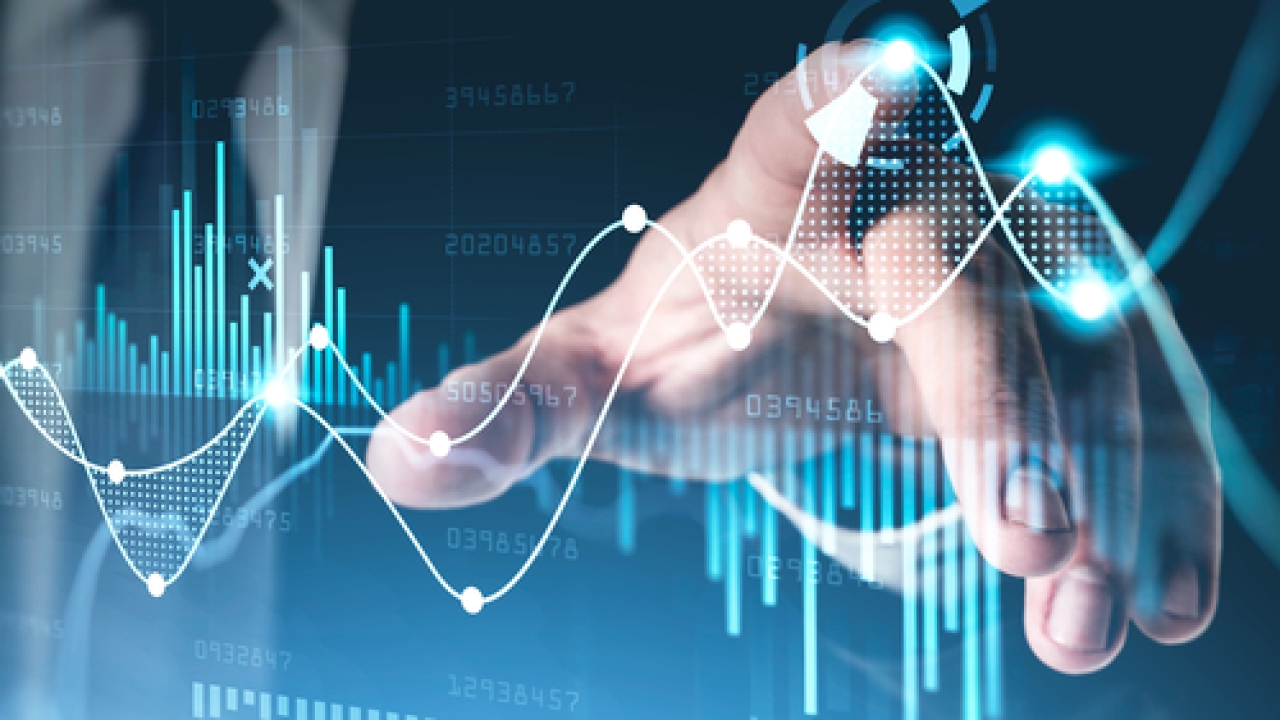
Anomaly Detection: ML algorithms can analyze network traffic patterns and identify anomalous behavior indicative of security threats, performance issues, or infrastructure faults, enabling proactive detection and mitigation of network abnormalities.
Predictive Analytics: ML network monitoring can analyze historical network data to predict future network performance, capacity requirements, and potential failures, allowing IT teams to anticipate and address issues before they impact operations.
Automated Remediation: ML-powered network monitoring systems can automate routine tasks such as configuration management, device provisioning, and incident response, reducing manual intervention and accelerating problem resolution.
Optimized Performance: ML algorithms can optimize network performance by dynamically adjusting network configurations, traffic routing, and resource allocation based on real-time data and changing network conditions.
Enhanced Security: ML-based network monitoring solutions can detect and mitigate security threats such as malware, DDoS attacks, and insider threats by analyzing network traffic patterns, identifying suspicious behavior, and triggering immediate response actions.
Scalability and Flexibility: ML algorithms can scale to analyze large volumes of network data from diverse sources, including IoT devices, cloud services, and virtualized environments, making them suitable for enterprise-scale network monitoring.
ML Techniques Used in Network Monitoring
Anomaly Detection: ML algorithms such as clustering, classification, and time series analysis are used to identify deviations from normal network behavior, flagging suspicious activities and potential security incidents.
Predictive Maintenance: ML models leverage regression analysis, time series forecasting, and machine learning algorithms to predict network failures, equipment malfunctions, and performance degradation, enabling proactive maintenance and resource allocation.
Pattern Recognition: ML algorithms analyze network traffic patterns, user behavior, and application performance metrics to identify recurring patterns and trends, facilitating capacity planning, traffic optimization, and network optimization.
Behavioral Analysis: ML techniques such as supervised learning, unsupervised learning, and reinforcement learning are used to analyze user behavior, device interactions, and application performance, identifying deviations from normal behavior and flagging potential security risks.
Natural Language Processing (NLP): NLP algorithms analyze unstructured data sources such as network logs, error messages, and incident reports, extracting valuable insights and contextual information to support decision-making and problem resolution.
Use Cases of ML in Network Monitoring
Security Threat Detection: ML-powered network monitoring systems can detect and respond to security threats such as malware, ransomware, and phishing attacks by analyzing network traffic patterns, identifying suspicious behavior, and triggering automated response actions.
Performance Optimization: ML algorithms can optimize network performance by dynamically adjusting network configurations, traffic routing, and resource allocation based on real-time data and changing network conditions.
Predictive Maintenance: ML models can predict network failures, equipment malfunctions, and performance degradation, enabling proactive maintenance and resource allocation to minimize downtime and service disruptions.
Capacity Planning: ML-based network monitoring solutions can analyze historical data and predict future network capacity requirements, enabling organizations to scale their infrastructure and resources to meet growing demand and avoid performance bottlenecks.
User Experience Optimization: ML algorithms can analyze user behavior, application performance, and network latency to identify factors affecting user experience and recommend optimizations to improve overall satisfaction and productivity.
In summary, ML-powered network monitoring offers organizations a powerful tool for proactively managing and optimizing their network infrastructure, enhancing security, reliability, and performance while reducing operational overhead and downtime. By leveraging advanced analytics and automation, organizations can stay ahead of emerging threats, predict and prevent network issues, and deliver a seamless and secure network experience for users.
For more info. visit us:
https://latestsms.in/funny-good-morning-sms.htm
24 July, 2024
https://www.cargoes.com/rostering-system
23 November, 2022
https://www.cargoes.com/rostering-system
88434 Views
http://aptrondelhi.in/
26385 Views
http://thetradieaccountant.com.au/
4 August, 2017
http://www.aluminumalloyformwork.com/
18 April, 2017